Design of Experiments for Optimisation

- Descrição
- Currículo
- FAQ
- Revisões
Welcome to “Design of Experiments for Optimisation“!
Experimentation plays an important role in science, technology, product design and formulation, commercialization, and process improvement. A well-designed experiment is essential once the results and conclusions that can be drawn from the experiment depend on the way the data is collected.
This course will cover the basic concepts behind the Response Surface Methodology and Experimental Designs for maximising or minimising response variables.
This is not a beginner course, so to get the most of it, you need to be familiar with some basic concepts underlying the design of experiments, such as analysis of variance and factorial designs.
You can find it in my course “Design and Analysis of Experiments” or on several other courses and resources on the market.
The course starts with a basic introduction to linear regression models and how to build regression models to fit experimental data and check the model adequacy. The next section covers experimental designs for linear models and the use of central points to check the model’s linearity (lack-of-fit). By the end of the section, we will be using linear models to fit experiments with inaccurate levels in the design factors and missing observations.
By then, we will be ready for Response Surface Methodology. We will start with a factorial design to fit a linear model and find the path of the steepest ascent. And then, we are going to use a central composite design to fit a quadratic model and find the experimental conditions that maximise the response. Moreover, we will see how to analyse several responses simultaneously using two very illustrative and broad examples.
Finally, we will see how to use three-level designs: Box-Behnken and face-centred composite designs.
The whole learning process is illustrated with real examples from research in the industry and in the academy.
The analysis of the data will be performed using R-Studio. Although this is not an R course, even students who are not familiar with R can enrol in it. The R codes and the data files used in the course can be downloaded, the functions will be briefly explained, and the codes can be easily adapted to analyse the student’s own data.
However, if you are already familiar with using other DoE software, feel free to download the data and reproduce the analysis using the software of your choice. The results will be exactly the same.
Any person who performs experiments can benefit from this course, mainly researchers from the academy and the industry, Master and PhD students and engineers.
-
3Introduction to Linear Regression ModelsVídeo Aula
-
4Fitting Linear Regression Models to Experimental DataVídeo Aula
-
5Building Linear Models in RVídeo Aula
-
6Analysis of Variance of the Regression ModelVídeo Aula
-
7Residuals PlotsVídeo Aula
-
8Visualisation and Interpretation of the ResultsVídeo Aula
-
9Multiple Linear Regression ModelsVídeo Aula
-
10Multiple Linear Regression Models for Designed ExperimentsVídeo Aula
-
11Designs for Linear Models - 2ˆk DesignVídeo Aula
-
12Adding Central Points to the 2ˆk DesignVídeo Aula
-
13Building a 2ˆ3 design with central points in RVídeo Aula
-
14Analysing a 2ˆ3 design with central points in RVídeo Aula
-
15Interpreting the resultsVídeo Aula
-
16Designs with missing observations and inaccurate levels of design factorsVídeo Aula
-
17Solving a design with missing observations and inaccurate levelsVídeo Aula
-
18Introduction to Response Surface MethodologyVídeo Aula
-
19The Starting DesignVídeo Aula
-
20Analysing the First DesignVídeo Aula
-
21The Method Steepest AscentVídeo Aula
-
22Interpreting Lousy FittingVídeo Aula
-
23The Central Composite DesignVídeo Aula
-
24Building a Central Composite Design in RVídeo Aula
-
25Analysing a Central Composite Design in RVídeo Aula
-
26Analysing Multiple Responses Simultaneously (1)Vídeo Aula
-
27Analysing Multiple Responses Simultaneously (2)Vídeo Aula
-
28Desirability Functions for Optimising Multiple ResponsesVídeo Aula
-
29Desirability Functions - R tutorialVídeo Aula
-
30Central Composite Design for 3 FactorsVídeo Aula
-
31Case Study: The Starbucks Coffee Bag - 1Vídeo Aula
-
32Case Study: The Starbucks Coffee Bag - 2Vídeo Aula
-
33Case Study: The Starbucks Coffee Bag - R tutorial - Adding the Coded VariablesVídeo Aula
-
34Case Study: The Starbucks Coffee Bag - Analysing the Tear ResultsVídeo Aula
-
35Case Study: The Starbucks Coffee Bag - Analysing the Leakage ResultsVídeo Aula
-
36Case Study: The Starbucks Coffee Bag - Final Interpretation of the ResultsVídeo Aula
-
376.H Applying Desirability functions to The Starbucks Coffee Bag Case StudyVídeo Aula
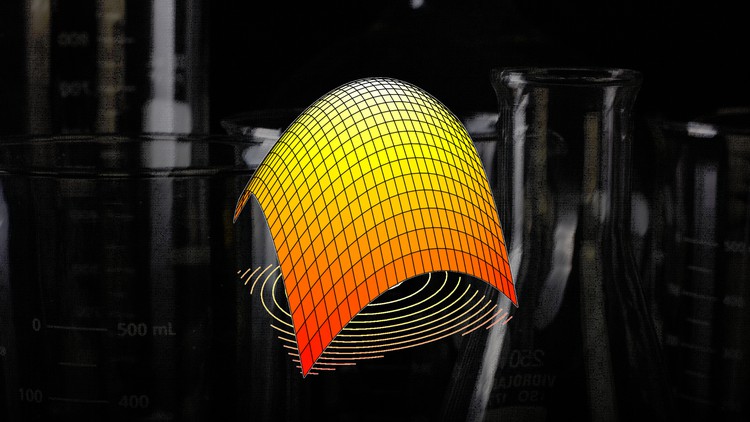