Master statistics & machine learning: intuition, math, code
- Descrição
- Currículo
- FAQ
- Revisões
Statistics and probability control your life. I don’t just mean What YouTube’s algorithm recommends you to watch next, and I don’t just mean the chance of meeting your future significant other in class or at a bar. Human behavior, single-cell organisms, Earthquakes, the stock market, whether it will snow in the first week of December, and countless other phenomena are probabilistic and statistical. Even the very nature of the most fundamental deep structure of the universe is governed by probability and statistics.
You need to understand statistics.
Nearly all areas of human civilization are incorporating code and numerical computations. This means that many jobs and areas of study are based on applications of statistical and machine-learning techniques in programming languages like Python and MATLAB. This is often called ‘data science’ and is an increasingly important topic. Statistics and machine learning are also fundamental to artificial intelligence (AI) and business intelligence.
If you want to make yourself a future-proof employee, employer, data scientist, or researcher in any technical field — ranging from data scientist to engineering to research scientist to deep learning modeler — you’ll need to know statistics and machine-learning. And you’ll need to know how to implement concepts like probability theory and confidence intervals, k-means clustering and PCA, Spearman correlation and logistic regression, in computer languages like Python or MATLAB.
There are six reasons why you should take this course:
-
This course covers everything you need to understand the fundamentals of statistics, machine learning, and data science, from bar plots to ANOVAs, regression to k-means, t-test to non-parametric permutation testing.
-
After completing this course, you will be able to understand a wide range of statistical and machine-learning analyses, even specific advanced methods that aren’t taught here. That’s because you will learn the foundations upon which advanced methods are build.
-
This course balances mathematical rigor with intuitive explanations, and hands-on explorations in code.
-
Enrolling in the course gives you access to the Q&A, in which I actively participate every day.
-
I’ve been studying, developing, and teaching statistics for over 20 years, and I think math is, like, really cool.
What you need to know before taking this course:
-
High-school level maths. This is an applications-oriented course, so I don’t go into a lot of detail about proofs, derivations, or calculus.
-
Basic coding skills in Python or MATLAB. This is necessary only if you want to follow along with the code. You can successfully complete this course without writing a single line of code! But participating in the coding exercises will help you learn the material. The MATLAB code relies on the Statistics and Machine Learning toolbox (you can use Octave if you don’t have MATLAB or the statistics toolbox). Python code is written in Jupyter notebooks.
-
I recommend taking my free course called “Statistics literacy for non-statisticians“. It’s 90 minutes long and will give you a bird’s-eye-view of the main topics in statistics that I go into much much much more detail about here in this course. Note that the free short course is not required for this course, but complements this course nicely. And you can get through the whole thing in less than an hour if you watch if on 1.5x speed!
-
You do not need any previous experience with statistics, machine learning, deep learning, or data science. That’s why you’re here!
Is this course up to date?
Yes, I maintain all of my courses regularly. I add new lectures to keep the course “alive,” and I add new lectures (or sometimes re-film existing lectures) to explain maths concepts better if students find a topic confusing or if I made a mistake in the lecture (rare, but it happens!).
You can check the “Last updated” text at the top of this page to see when I last worked on improving this course!
What if you have questions about the material?
This course has a Q&A (question and answer) section where you can post your questions about the course material (about the maths, statistics, coding, or machine learning aspects). I try to answer all questions within a day. You can also see all other questions and answers, which really improves how much you can learn! And you can contribute to the Q&A by posting to ongoing discussions.
And, you can also post your code for feedback or just to show off — I love it when students actually write better code than me! (Ahem, doesn’t happen so often.)
What should you do now?
First of all, congrats on reading this far; that means you are seriously interested in learning statistics and machine learning. Watch the preview videos, check out the reviews, and, when you’re ready, invest in your brain by learning from this course!
-
1[Important] Getting the most out of this courseVídeo Aula
Strategies for optimal learning.
-
2About using MATLAB or PythonVídeo Aula
How to use different programming languages in the course.
-
3Statistics guessing game!Vídeo Aula
Simulate data and run a statistical analysis. A fun way to start the course :)
-
4Using the Q&A forumVídeo Aula
I explain how to get the most out of the interactive part of this course: The Q&A forum!
-
5(optional) Entering time-stamped notes in the Udemy video playerVídeo Aula
-
6Should you memorize statistical formulas?Vídeo Aula
A discussion about memorizing formulas.
-
7Arithmetic and exponentsVídeo Aula
A reminder about foundational arithmetic rules.
-
8Scientific notationVídeo Aula
Ways of representing very large and very small numbers.
-
9Summation notationVídeo Aula
Mathematical notation for adding a series of numbers.
-
10Absolute valueVídeo Aula
Absolute value is the distance away from zero, regardless of sign.
-
11Natural exponent and logarithmVídeo Aula
Natural exponent and logarithm are two of the most important functions in math and its applications.
-
12The logistic functionVídeo Aula
The logistic function is used often in statistics, machine learning, and optimization.
-
13Rank and tied-rankVídeo Aula
To rank data means to transform raw numerical values into ordinal position. Rank is used in non-parametric statistics.
-
15Is "data" singular or plural?!?!!?!Vídeo Aula
My take on statistical terminology, grammar, and modern culture.
-
16Where do data come from and what do they mean?Vídeo Aula
A philosophical discussion about how we can obtain numbers from the universe.
-
17Types of data: categorical, numerical, etcVídeo Aula
Data come in different forms, which has implications for ways of visualizing and analyzing data.
-
18Code: representing types of data on computersVídeo Aula
Introduction to data types in MATLAB and Python.
-
19Sample vs. population dataVídeo Aula
There is an important distinction between measuring *all* of the data vs. some of the data.
-
20Samples, case reports, and anecdotesVídeo Aula
This distinction is related to sample size, and has implications for the generalizability of experimental findings.
-
21The ethics of making up dataVídeo Aula
The take-home message here is simple: Don't lie or cheat!
-
22Bar plotsVídeo Aula
Lecture on how to create and interpret bar plots, including the types of data that are used.
-
23Code: bar plotsVídeo Aula
Creating bar plots in MATLAB and Python, including parameters.
-
24Box-and-whisker plotsVídeo Aula
Creating and interpreting box plots, also called box-and-whisker plots.
-
25Code: box plotsVídeo Aula
Box plots in MATLAB and Python.
-
26"Unsupervised learning": Boxplots of normal and uniform noiseVídeo Aula
An exercise on creating box plots of random numbers drawn from different distributions.
-
27HistogramsVídeo Aula
A lecture on how to create and interpret histograms, including frequency vs. proportion.
-
28Code: histogramsVídeo Aula
Creating and visualizing histograms in code.
-
29"Unsupervised learning": Histogram proportionVídeo Aula
An exercise on transforming frequencies (counts) into proportions.
-
30Pie chartsVídeo Aula
Pie charts are nice visualizations when your data add up to 100%.
-
31Code: pie chartsVídeo Aula
Create pie charts in code. It's easier than you think!
-
32When to use lines instead of barsVídeo Aula
A critical discussion of how to visualize categorical vs. continuous data using lines vs. bars.
-
33Linear vs. logarithmic axis scalingVídeo Aula
A comparison of scaling the y-axis and x-axis intervals.
-
34Code: line plotsVídeo Aula
More on plotting and parameterizing line plots in code.
-
35"Unsupervised learning": log-scaled plotsVídeo Aula
An exercise on scaling data in different ways.
-
36Descriptive vs. inferential statisticsVídeo Aula
The term "statistics" actually has two broad meanings: characteristics of a sample vs. generalizing to other samples.
-
37Accuracy, precision, resolutionVídeo Aula
These terms relate to how your data relate to the real world objects that the data measure.
-
38Data distributionsVídeo Aula
Data come in different distributions, which has implications for how to visualize and analyze datasets.
-
39Code: data from different distributionsVídeo Aula
You will learn how to create random data with different distributions in MATLAB and Python.
-
40"Unsupervised learning": histograms of distributionsVídeo Aula
What happens when you plot the distribution of a distribution function? Find out!
-
41The beauty and simplicity of NormalVídeo Aula
The Gaussian distribution describes a remarkable and fundamental quality of the universe.
-
42Measures of central tendency (mean)Vídeo Aula
The mean, aka average, is the most common and insightful measure of a data set.
-
43Measures of central tendency (median, mode)Vídeo Aula
The mean is not appropriate for all data distributions; here you will learn two non-parametric measures of dataset centrality.
-
44Code: computing central tendencyVídeo Aula
Computing mean, median, and mode in MATLAB and Python.
-
45"Unsupervised learning": central tendencies with outliersVídeo Aula
An exercise to help you understand the impact of outliers on mean, median, and mode.
-
46Measures of dispersion (variance, standard deviation)Vídeo Aula
You will learn about dispersion, which is how wide the data distribution is.
-
47Code: Computing dispersionVídeo Aula
Computing different measures of dispersion in code.
-
48Interquartile range (IQR)Vídeo Aula
IQR is a measures of the spread of most (but not all) of the data, and is robust to outliers.
-
49Code: IQRVídeo Aula
See how to generate the interquartile range in code.
-
50QQ plotsVídeo Aula
QQ plots show how your data compare to a theoretical normal (Gaussian) distribution.
-
51Code: QQ plotsVídeo Aula
Learn how QQ plots are created in Python and MATLAB.
-
52Statistical "moments"Vídeo Aula
Moments are statistical characteristics of the data. Here you'll learn the first four moments of a distribution.
-
53Histograms part 2: Number of binsVídeo Aula
More on histograms: Learn the formulas for determining the number of bins (data discretizations) to use.
-
54Code: Histogram binsVídeo Aula
Experiment with histogram parameters.
-
55Violin plotsVídeo Aula
Learn how to create and interpret a beautiful graph for visualizing data and data distributions.
-
56Code: violin plotsVídeo Aula
See how violin plots are created in code. Tip: Use lots of colors!
-
57"Unsupervised learning": asymmetric violin plotsVídeo Aula
An exercise to visualize two data distributions in one violin plot.
-
58Shannon entropyVídeo Aula
Learn how to interpret this nonlinear measure of data dispersion.
-
59Code: entropyVídeo Aula
Shannon entropy in code.
-
60"Unsupervised learning": entropy and number of binsVídeo Aula
You will see how the bin-count parameter affects entropy.
-
61Garbage in, garbage out (GIGO)Vídeo Aula
No amount of fancy statistics or data cleaning can fix terrible data. Start with good data!
-
62Z-score standardizationVídeo Aula
Z-score is the most important data normalization in statistics and machine learning.
-
63Code: z-scoreVídeo Aula
Translate the z-score formula into code.
-
64Min-max scalingVídeo Aula
Min-max scaling is the second-most important data normalization method.
-
65Code: min-max scalingVídeo Aula
Translate min-max scaling into Python and MATLAB code.
-
66"Unsupervised learning": Invert the min-max scalingVídeo Aula
An exercise to get from normalized data back to their original scale.
-
67What are outliers and why are they dangerous?Vídeo Aula
Outliers are unusual values that can completely screw up your analyses and interpretation!
-
68Removing outliers: z-score methodVídeo Aula
This is one of the most common methods for identifying and removing outliers.
-
69The modified z-score methodVídeo Aula
The modified z-score method uses the median instead of the mean, and therefore is good for removing outliers in non-normal distributions.
-
70Code: z-score for outlier removalVídeo Aula
Implement the modified z-score method in code.
-
71"Unsupervised learning": z vs. modified-zVídeo Aula
Does it really matter if you use the regular or modified z-score method? Come find out!
-
72Multivariate outlier detectionVídeo Aula
Extend the z-score method to outliers in high-dimensional datasets.
-
73Code: Euclidean distance for outlier removalVídeo Aula
Multivariate outlier identification and removal, using concepts from geometry.
-
74Removing outliers by data trimmingVídeo Aula
Another common method for removing outliers, based on threshold-exceedance.
-
75Code: Data trimming to remove outliersVídeo Aula
See how data trimming is implemented in MATLAB and Python.
-
76Non-parametric solutions to outliersVídeo Aula
Instead of removing outliers, you can use analyses that are robust to outliers.
-
77Nonlinear data transformationsVídeo Aula
Some outliers can be transformed into non-outliers by applying certain nonlinear transformations.
-
78An outlier lecture on personal accountabilityVídeo Aula
A lecture on one of the main challenges of online learning. Just something to reflect on.
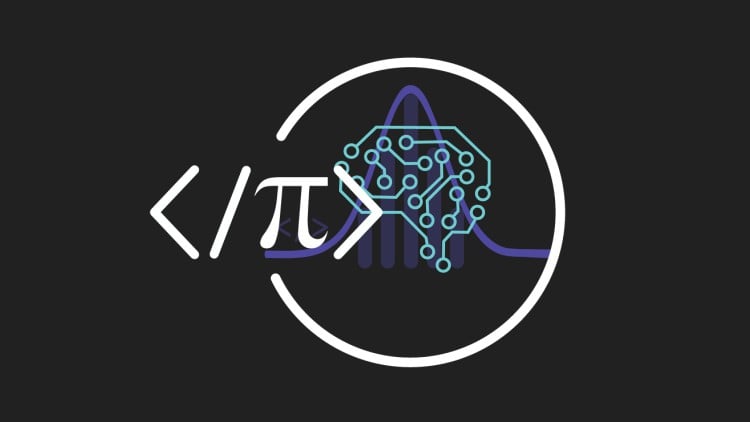